Accessibility
Project Euphonia: Making Speech Recognition Accessible to All
Voice technology, be it digital assistants, real-time translation, or smart tech, is having a growing impact on everyday life. But where does this leave those for whom voice technology is not accessible? Ellen Daniel spoke to one of the people addressing this through Google’s Project Euphonia to uncover more
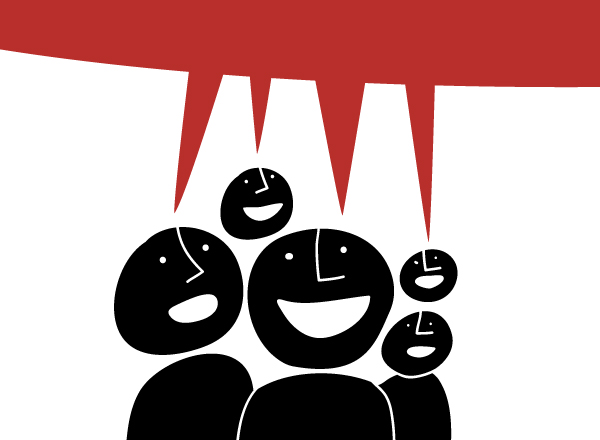
Technological innovation has the potential to make the world more accessible, with devices such as hands-free mice, one-handed keyboards, screen readers and voice search making it easier for those with disabilities to carry out their jobs and bridge barriers in communication.
This trend has extended to smart home technology, which has the potential to assist those with disabilities or additional needs, enabling them to carry out everyday tasks more easily.
However, for some, the rapid development of technology risks presenting a barrier rather than an opportunity. Those with non-standard speech, which can be caused by neurologic conditions such as strokes, ALS, multiple sclerosis, traumatic brain injuries and Parkinson's, may face barriers when it comes to interacting with such devices.
Therefore, a number of organisations are working towards developing the next generation of accessible technology, powered by AI.
Accessibility and speech recognition: Inclusivity by design
According to Juniper Research, the use of digital voice assistants is expected to triple to eight billion by 2023, with smart home devices such as smart TVs, smart speakers and wearables.
Hector Minto, Accessibility Lead for Microsoft EMEA, told Verdict that it is essential that technological advances have accessibility at their heart:
“There’s an opportunity to more inclusively design when you recognise the people that are excluded from technology as it stands today.”
“As people move to the cloud and as people start to involve AI, there’s a huge opportunity to make sure that technology is more routinely inclusive because, if you think about AI as technology that can hear, can see, can think, can speak, and then frame that around disability, it offers a huge amount.”
“There’s an opportunity to more inclusively design when you recognise the people that are excluded from technology as it stands today.”
One organisation working to make speech technology more universally accessible is Google. The company has developed Project Euphonia to make voice technology more accessible.
Euphonia performs speech-to-text transcription and is trained using a personalised speech dataset from those with non-standard speech, particularly those with ALS.
Automatic speech recognition systems are typically trained using standard speech, meaning that certain groups may be underrepresented if they are not included in the datasets it is trained on, but if they are trained to recognise individuals’ speech, barriers of communication with both people and voice assistants can be broken down.
Breaking down communication barriers
Research scientist Dimitri Kanevsky has played a key role in Google’s advances in accessible speech recognition. Kanevsky lost his hearing at the age of one, and has been in the speech recognition field for thirty years. He explained that his work stems from difficulties in communicating he faced earlier in his career:
“I lipread well in Russian…but it became a big problem in America. They had no transcription services so it was very difficult to communicate. [When I was working] at IBM, I developed the first communication access real-time translation (CART) services in the world over the internet.
“The internet had just started and somebody showed me Webex. Webex allows communication between two computers in different locations. When I saw this, I immediately called one of my court reporters and said ‘call my office, install Webex on your computer and type everything you hear’. They did this and it was the start of CART internet services.”
The development of accessible speech recognition
Kanevsky explained that at the beginning of his work on speech recognition technology, he anticipated that a solution would be reached far sooner than it was:
“I understood that I needed to focus on developing more speech recognition technology...I thought that we'd develop speech recognition very quickly and that in five years we'd have a full solution for this. And in five years I thought it would take another five years. But it went for 25 years! Speech recognition became better and better but it still wasn't good enough.”
Kanevsky has trained voice-recognition technology developed through the project to recognise his own speech, based on recordings of many different phrases:
“The model that you can see now is based on 25 hours of recordings...I started using Euphonia after a small amount of recorded speech. First I recorded speech to communicate with Google Home. You can record 100 commands like “make me laugh” or “what is the weather today?” You can record, train and it immediately becomes useful.”
“I thought that we'd develop speech recognition very quickly and that in five years we'd have a full solution for this.”
In his case, this meant training the tool to interpret his voice when he gave lectures:
“I recorded my lectures…at the beginning I was speaking exactly like I trained it. If I would change [what I was saying] it would make errors. But after I recorded more and more lectures, it started to understand phrases that I did not record. So eventually it started to understand anything that I said. So now I don’t need to train it again.”
Not only has this enabled better communication for Kanevsky in a professional capacity, it has also helped him communicate with his granddaughters.
Kanevsky explained that real-time translation services can be prohibitively expensive for many businesses, limiting the roles or organisations those who rely on them can work in.
“The CART companies created services for a lot of people…but they were very expensive. So only big companies like IBM or Google could afford this. So I was constrained to only work at big companies that can afford this. But now I have full freedom.
“If we had met more than one year ago, our conversation would’ve cost Google a few thousand dollars.”
Training algorithms
Speech recognition software is typically trained using many hours of speech, which presents issues when the algorithm is being trained to recognise a single voice.
Under its AI for Good initiative, Google has partnered with ALS Therapy Development Institute (ALS TDI) and ALS Residence Initiative (ALSRI) to record the voices of people who have ALS and optimise “AI-based algorithms so that mobile phones and computers can more reliably transcribe words spoken by people with these kinds of speech difficulties”.
This could enable individuals to compose text-based messages using voice and interact with voice assistants.
Kanevsky hopes that a tool can be developed that will recognise non-standard speech without every person having to extensively train it:
“For people with ALS, we can use recordings from many people that have similar [speech] patterns. So we have an acoustic model for many people with ALS from recordings of a number of phrases. So the acoustic model can better understand everybody. We hope that if a lot of people record their voice, that if a new person [uses it] they do not have to train it again. It will only have to be trained a little bit.”
“For people with ALS, we can use recordings from many people that have similar speech patterns.”
Kanevsky explained that obtaining enough data in order to develop algorithms has been a barrier, but in recent years, researchers have been able to utilise an unlikely source:
“Live Transcribe is public, free, available in 70 languages and anybody can download it in Android. Euphonia is a research project that’s only available for people who work for Google…I feel that we still need to collect a lot of data for different types of speech variations, but if we have enough clusters, it starts to match [different speech].
“I think the biggest challenge was developing algorithms that understand speech recognition. When the algorithms were done, the application was developed quickly. The biggest progress in developing algorithms came with neural networks.
“The concept of neural networks existed for 30 years but a few years ago it started to show good results because computers became fast enough to run very complex systems. Another factor that helped in developing speech recognition was YouTube. YouTube has a lot of videos where people put manual transcriptions. So you get a lot of data for free to train speech recognition. Before, to train speech recognition you hired transcribers. It’s very expensive and slow.”
An additional option, not a replacement
He hopes that Euphonia will make it easier for those with non-standard speech to interact with both technology and other people:
“Euphonia needs to become such that every person who has non-standard speech who wants to communicate with voice interactive devices like Google Home or Google Assistant, who want to communicate with people, could just go on a website, record their voice, or find a model that already fits their voice, put on their device and use.”
“We’re not proposing a system that stops the use of sign language. It’s in addition to sign language.”
However, Kanevsky emphasised that tools such as Euphonia are not intended to replace other methods of communication, such as sign language, but offer additional options for individuals to utilise:
“We’re not proposing a system that stops the use of sign language. It’s in addition to sign language. At Google we do a lot of work to develop systems that understand sign language so eventually I think that people will be able to both.”
Back to top